Self-Driving Network Researcher Arpit Gupta Joins Berkeley Lab’s Scientific Networking Division as a Faculty Scientist
By Bonnie Powell, bpowell@es.net
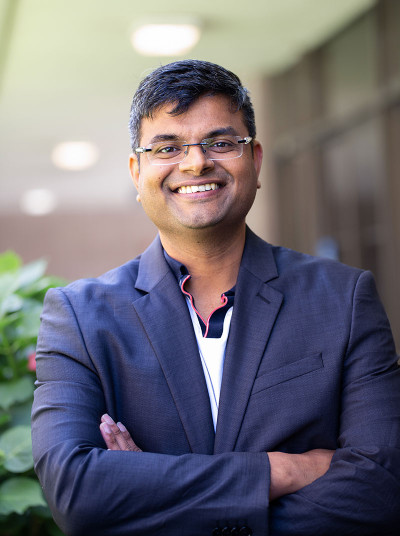
Arpit Gupta is an Assistant Professor of Computer Science at UC Santa Barbara and codirector of UCSB’s Systems and Networking Lab. He joins the Scientific Networking Division as a faculty scientist.
The Scientific Networking Division of Lawrence Berkeley National Laboratory’s Computing Sciences Area is pleased to announce the appointment of networking researcher Arpit Gupta, Assistant Professor of Computer Science at UC Santa Barbara and co-director of UCSB’s Systems and Networking Lab, as faculty scientist. At Berkeley Lab, Gupta will continue his groundbreaking work developing and validating foundation models for use in networking to build the “self-driving networks” of the future.
“Foundation models” are AI models trained on broad types of data, are generally self-supervised in their learning, contain at least tens of billions of parameters, and can be applied across a wide range of contexts. OpenAI’s GPT is the best-known example of a foundation model.
Gupta will work closely with staff from the Planning & Innovation Department of Energy Sciences Network (ESnet), the Department of Energy’s high-performance network for scientific research, which in 2023 transported 1.7 exabytes of data. Gupta’s models have the potential to unlock valuable insights from ESnet’s massive cache of monitoring data, gathered through its High Touch telemetry project and enabled by the advanced design of ESnet6. The researchers will also be leveraging the processing power of the Perlmutter supercomputer at the National Energy Research Scientific Computing Center (NERSC), which, like ESnet, is stewarded by Berkeley Lab.
Gupta’s research focuses on developing production-ready ML artifacts for networking that are both performant and generalizable in target production settings. He has developed two software tools, netUnicorn and Trustee, for this purpose: netUnicorn simplifies the collection of training data for various learning problems and network environments, while Trustee helps users understand a model's decision-making process. Together, they enable an analysis-driven ML workflow that iteratively curates the "right" training data, free from biases and skews, to improve model generalizability.
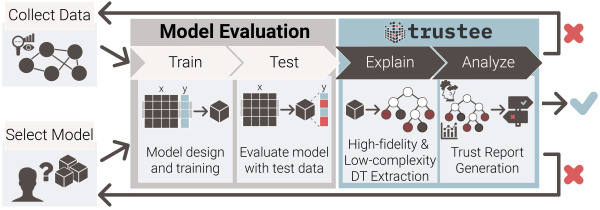
A flowchart of the standard AI/ML development pipeline extended by Trustee, a framework that Gupta's team developed to extract decision-tree explanations from black-box ML models
More recently, Gupta’s team has developed netFound, a network foundation model designed to leverage the unique characteristics of networking data, such as protocol semantics, inherent hierarchy, and multi-modality. This model uses self-supervised learning techniques to uncover hidden spatial and temporal relationships in abundant, unlabeled telemetry data from production networks. The pre-trained foundation model can then be fine-tuned for specific learning problems and network environments using labeled data curated through the closed-loop ML workflow, resulting in performant, robust, and generalizable ML models.
“I have long been inspired by ESnet leadership’s abilities to foresee the transformative potential of new ideas — for example, the development of its state-of-the-art high-touch telemetry infrastructure. I am convinced that network foundation models will have a similarly transformative effect on networking as software-defined networking did in the past decade,” said Gupta. “Leveraging the untapped potential of abundant, yet unlabeled, telemetry data can liberate us from the limitations of sparse, noisy, and skewed datasets that have hindered us for decades. Working with NERSC, we hope to swiftly develop a robust and performant network foundation model and, more importantly, democratize its access for all. This model can be fine-tuned for numerous existing and unexplored learning tasks in networking, significantly advancing the goal of developing ‘self-driving networks’ that enable safe and performant network connectivity with minimal human intervention.”
This is not ESnet’s first foray into AI for networking, nor will it be its last. ESnet’s leadership has already begun looking ahead to ESnet7, the next iteration of the network, which is projected to be completed in the next three to five years. Artificial Intelligence for IT Operations, or AIOps, will be one of the key ways through which ESnet continues to transform and expand its networking capabilities.
“Professor Gupta’s innovative approaches, ESnet’s networking data, and NERSC’s compute power are a formidable combination,” said Inder Monga, director of ESnet and the Scientific Networking Division. “As a community, we’re on the brink of an AI revolution as exciting as the Internet. Just as we did then, we must collaborate on this kind of nonproprietary research to be ready for the complex challenges the future will bring.”